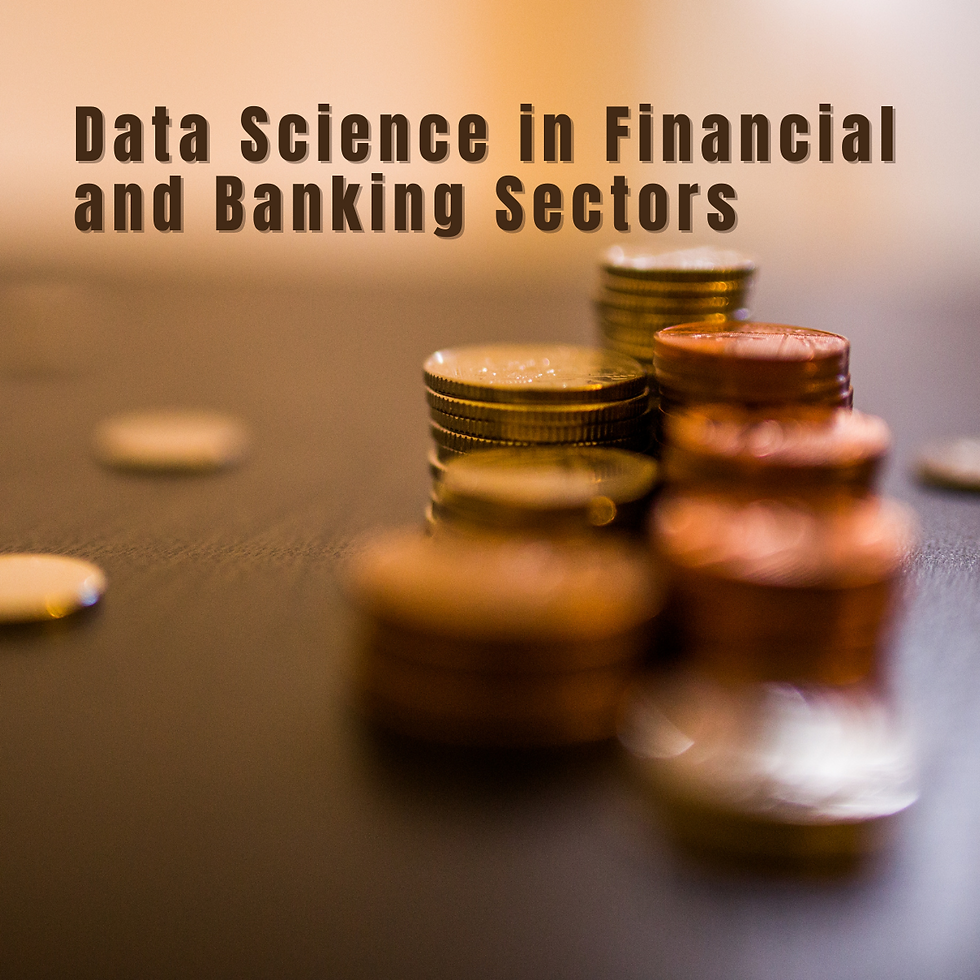
Introduction
Data science, a multidisciplinary field that combines statistics, computer science, and domain knowledge, has become a cornerstone of innovation in the financial and banking sectors. By leveraging large datasets and sophisticated algorithms, data science is revolutionizing the way banks and financial institutions operate, offering new avenues for efficiency, security, and customer satisfaction. This article delves into the critical role of data science in these sectors, highlighting its applications, benefits, challenges, and future prospects.
The Evolution of Data Science in Finance
Historical Context
The application of data analysis in banking dates back to the early days of computerized record-keeping. Initially, data processing in banks was limited to basic tasks like accounting and transaction recording. However, as computational power increased, so did the complexity and scope of data analysis. Financial modeling and algorithmic trading emerged in the late 20th century, driven by advancements in technology and the growing availability of financial data. The introduction of big data in finance marked a significant milestone, enabling the processing of massive datasets that were previously unmanageable.
Defining Data Science
Data science encompasses a range of techniques and methodologies designed to extract insights and knowledge from structured and unstructured data. Key components of data science include data mining, which involves discovering patterns in large datasets; machine learning, which allows systems to learn from data and make predictions; and predictive analytics, which uses historical data to forecast future trends. In the context of modern finance, data science is indispensable for making informed decisions, managing risks, and enhancing customer experiences.
Key Applications of Data Science in Banking
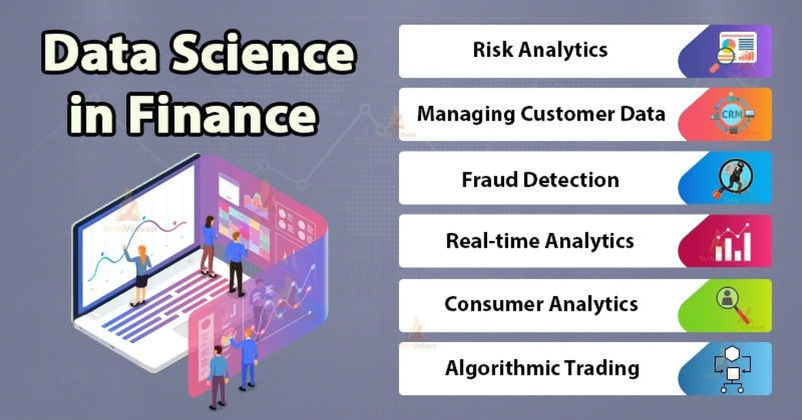
Fraud Detection and Prevention
Fraud detection is one of the most critical applications of data science in banking. By employing techniques such as anomaly detection and pattern recognition, banks can identify unusual transactions that may indicate fraudulent activity. For example, machine learning algorithms can analyze transaction patterns to detect deviations from typical behavior, flagging potentially fraudulent transactions for further investigation. Case studies have shown that banks utilizing these technologies can significantly reduce the incidence of fraud, protecting both their assets and their customers.
Risk Management
Risk management is another area where data science plays a vital role. Predictive analytics allows banks to assess credit risk more accurately by analyzing a wide range of factors, including credit history, transaction behavior, and economic indicators. Real-time risk monitoring systems provide continuous assessment of financial risks, enabling banks to respond swiftly to emerging threats. By leveraging data science, banks can make more informed lending decisions and maintain a healthier loan portfolio.
Customer Analytics
Data science enables banks to gain deeper insights into their customers' needs and preferences. By analyzing customer data, banks can segment their customer base and tailor services to individual needs, enhancing customer satisfaction. For instance, predictive models can identify which customers are likely to require certain financial products, allowing banks to offer personalized recommendations. Additionally, data science can improve customer service by powering AI-driven chatbots that provide instant, accurate responses to customer inquiries.
Algorithmic Trading
Algorithmic trading, which relies on automated systems to execute trades based on predefined criteria, is heavily dependent on data science. High-frequency trading (HFT) is a subset of algorithmic trading that uses complex algorithms to analyze market data and execute trades at extremely high speeds. Data science improves market efficiency and liquidity by enabling traders to make split-second decisions based on vast amounts of data. This technology has transformed trading, making it faster and more precise.
Regulatory Compliance
Financial institutions must comply with a myriad of regulations designed to ensure the stability and integrity of the financial system. Data science helps banks automate compliance processes by monitoring transactions for signs of non-compliance and generating reports for regulatory authorities. By integrating data science into their compliance frameworks, banks can reduce the risk of regulatory breaches and the associated penalties.
Credit Scoring
Traditional credit scoring methods, such as FICO scores, rely on a limited set of data points to assess creditworthiness. Data science allows for the development of more sophisticated credit scoring models that incorporate a wider range of variables, including social media activity, online behavior, and alternative financial data. These advanced models provide a more comprehensive assessment of an individual's credit risk, leading to better lending decisions and reduced default rates.
Loan and Investment Forecasting
Predictive models are invaluable for forecasting the performance of loans and investments. By analyzing historical data and market trends, data science can predict future loan defaults, enabling banks to adjust their lending strategies accordingly. Similarly, investment forecasting models help banks identify profitable investment opportunities and manage their portfolios more effectively.
Operational Efficiency
Data science enhances operational efficiency by automating routine tasks and streamlining banking processes. For example, robotic process automation (RPA) uses data-driven algorithms to perform repetitive tasks, such as processing loan applications or reconciling accounts. This reduces the time and cost associated with manual processes, allowing banks to allocate resources to more strategic activities.
Benefits of Data Science in the Financial Sector
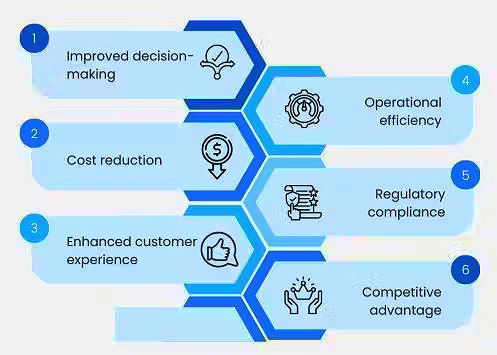
Enhanced Decision Making
Data-driven decision-making is one of the primary benefits of data science in finance. By leveraging real-time analytics, banks can make more informed decisions that are based on accurate and up-to-date information. This improves strategic planning and operational efficiency, ultimately leading to better financial outcomes.
Improved Security
With the increasing prevalence of cyber threats, data security is a top priority for financial institutions. Data science enhances cybersecurity measures by analyzing patterns in network traffic to detect potential threats and vulnerabilities. Advanced encryption techniques and secure transaction protocols, powered by data science, protect sensitive financial data from unauthorized access.
Increased Customer Satisfaction
Personalized banking experiences, enabled by data science, lead to higher levels of customer satisfaction. By analyzing customer behavior and preferences, banks can offer tailored products and services that meet individual needs. AI-driven chatbots and virtual assistants, which provide instant support and resolve issues efficiently, further enhance the customer experience.
Cost Reduction
Automation of routine tasks through data science reduces operational costs by minimizing the need for manual labor and reducing the likelihood of human error. For example, automated loan processing systems can handle applications more quickly and accurately than human employees, leading to faster approval times and lower processing costs.
Challenges and Limitations
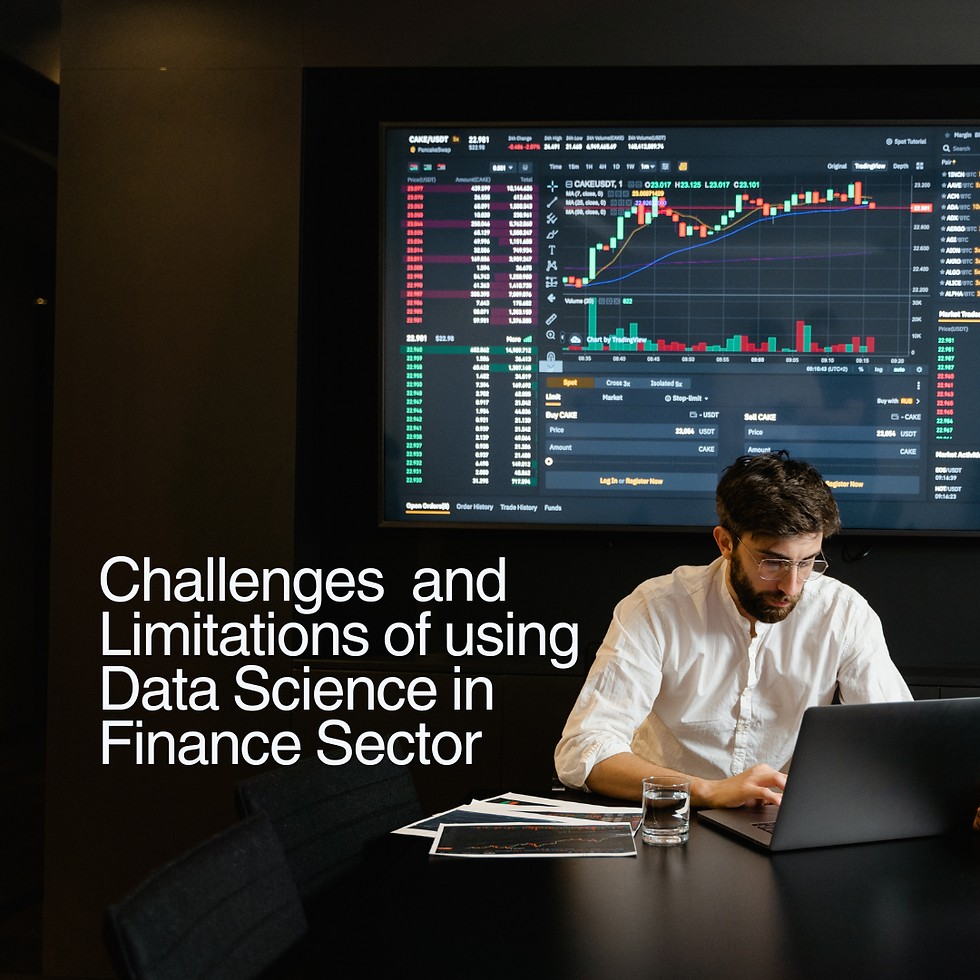
Data Privacy Concerns
While data science offers numerous benefits, it also raises significant privacy concerns. Financial institutions must balance the need to utilize data for analysis with the obligation to protect customer privacy. Compliance with regulations such as the General Data Protection Regulation (GDPR) is essential to avoid legal repercussions and maintain customer trust.
Data Quality Issues
The effectiveness of data science models depends on the quality of the underlying data. Inaccurate or incomplete data can lead to erroneous conclusions and poor decision-making. Financial institutions must invest in robust data management practices to ensure the accuracy, consistency, and completeness of their data.
Technological Barriers
Integrating new data science technologies with legacy systems can be challenging and costly. Financial institutions often face technological barriers when attempting to upgrade their infrastructure to support advanced data analytics. Ensuring seamless integration and minimizing disruption to existing operations requires careful planning and investment.
Skill Gap
There is a significant shortage of skilled data scientists in the financial sector. To fully leverage the potential of data science, financial institutions must invest in training and development programs to build a workforce capable of handling complex data analytics tasks. Collaboration with academic institutions and industry partners can help bridge the skill gap.
Latest Innovations in Data Science for Finance
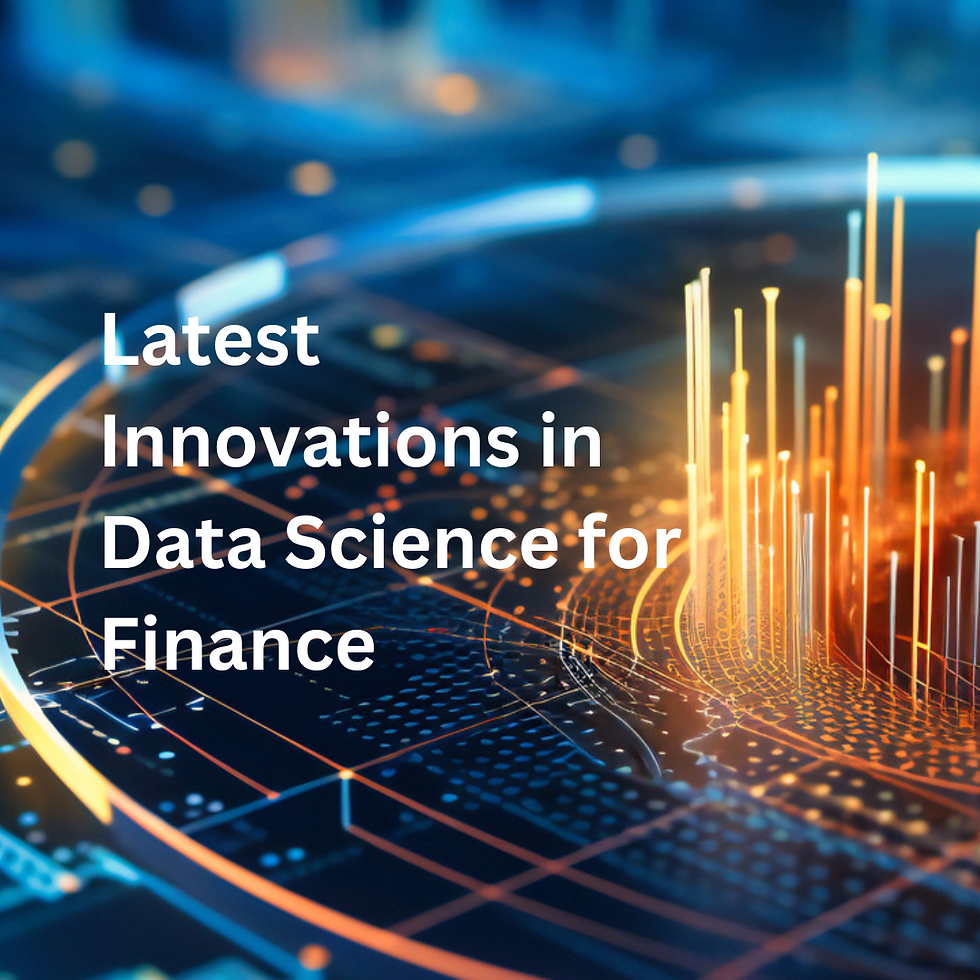
Artificial Intelligence and Machine Learning
Artificial intelligence (AI) and machine learning (ML) are at the forefront of data science innovations in finance. These technologies enable the development of predictive models that can analyze vast amounts of data to forecast trends and make informed decisions. AI-driven customer service tools, such as chatbots, enhance customer interactions by providing instant, accurate responses.
Blockchain and Data Science
Blockchain technology, known for its ability to create secure, transparent, and immutable records, is being integrated with data science to enhance financial transactions. By combining blockchain with data analytics, financial institutions can improve the security and transparency of transactions, reducing the risk of fraud and ensuring data integrity.
Quantum Computing
Quantum computing holds the potential to revolutionize financial modeling and risk analysis by performing complex calculations at unprecedented speeds. While still in its early stages, research into quantum computing for finance is progressing rapidly, with promising results that could transform the industry in the coming years.
Future Prospects
Emerging Trends
The future of data science in finance is marked by several emerging trends. Predictive and prescriptive analytics are expected to become more prevalent, enabling financial institutions to not only forecast future trends but also recommend optimal actions. The increasing use of real-time data will allow for more dynamic and responsive decision-making.
The Role of Fintech
Fintech companies are driving innovation in the financial sector by leveraging data science to develop new products and services. Collaboration between traditional banks and fintech startups is expected to increase, leading to the creation of innovative solutions that enhance financial services and improve customer experiences.
Regulatory Changes
As financial regulations continue to evolve, data science will play a crucial role in helping institutions comply with new requirements. Advanced analytics and automation will enable banks to adapt quickly to regulatory changes, ensuring ongoing compliance and reducing the risk of penalties.
Global Impact
Data science is not limited to developed markets; it is also making significant inroads in emerging economies. By providing access to financial services and insights, data science is helping to drive economic growth and financial inclusion in these regions. Cross-border financial analytics will become increasingly important as global markets become more interconnected.
Comparative Analysis
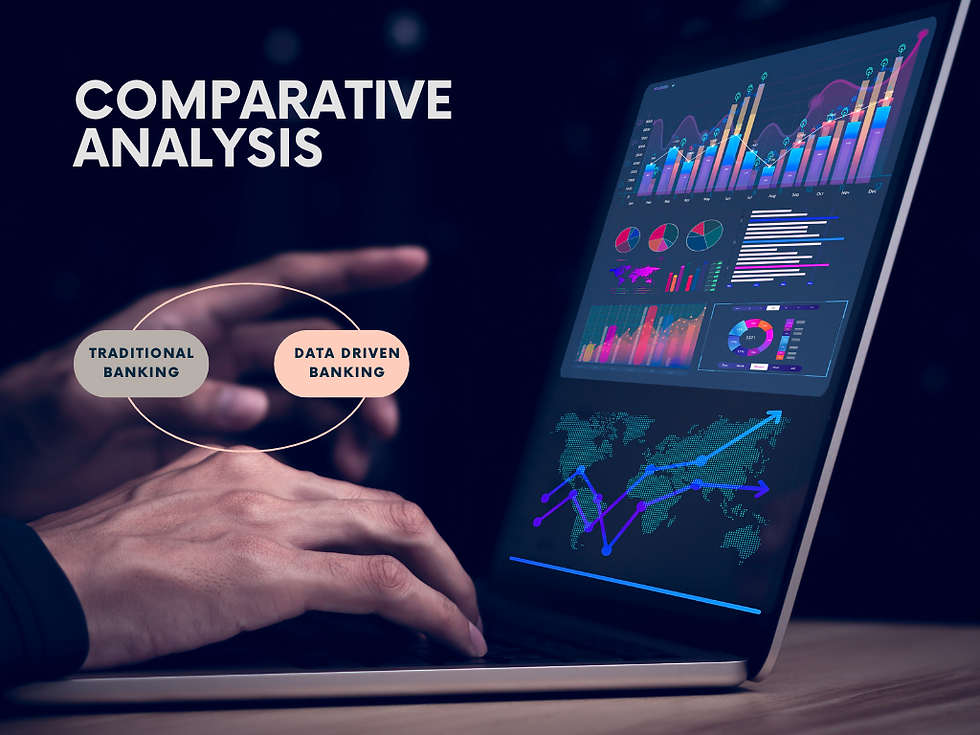
Traditional vs. Data-Driven Banking
Traditional banking relies heavily on manual processes and historical data, whereas data-driven banking leverages real-time analytics and automated systems. Data-driven banking offers several advantages, including improved efficiency, reduced costs, and enhanced decision-making capabilities. Case studies of banks that have adopted data science demonstrate significant improvements in performance and customer satisfaction.
Data Science in Different Financial Institutions
Data science applications vary across different types of financial institutions, such as banks, credit unions, and fintech companies. While banks may focus on risk management and fraud detection, fintech companies often prioritize customer analytics and personalized services. Each type of institution can benefit from data science in unique ways, tailored to their specific needs and goals.
User Guides and Tutorials
Implementing Data Science in Your Organization
To successfully implement data science in a financial institution, several steps must be taken:
Assess Needs: Identify the key areas where data science can add value.
Build Infrastructure: Invest in the necessary technology and tools.
Hire Experts: Recruit skilled data scientists and analysts.
Integrate Systems: Ensure seamless integration with existing systems.
Train Staff: Provide training and development programs for employees.
Building a Data Science Team
Building an effective data science team requires a clear understanding of the roles and responsibilities involved:
Hiring and training strategies should focus on attracting top talent and providing continuous learning opportunities to keep the team updated with the latest developments in data science.
Personal Stories and Case Studies
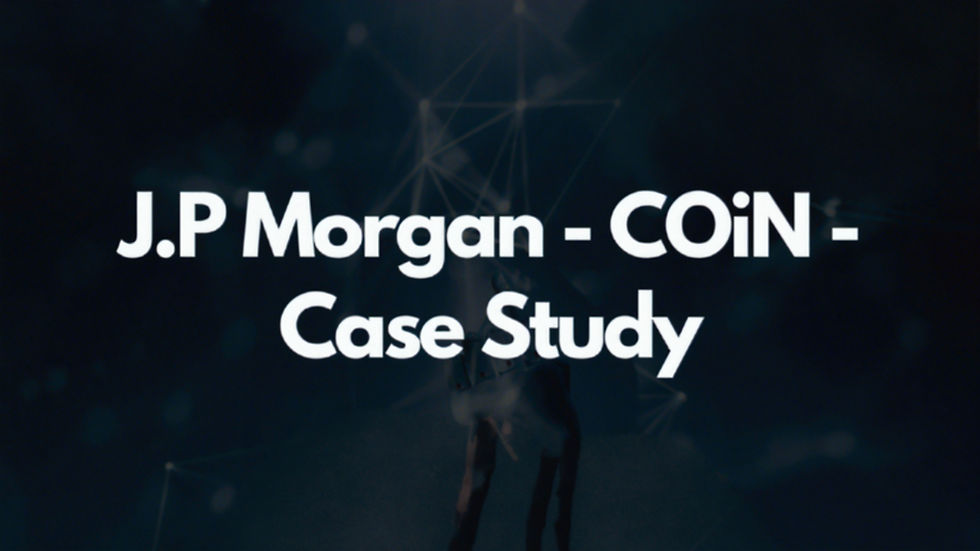
Success Stories
Several financial institutions have successfully implemented data science to transform their operations. For example, JPMorgan Chase has developed an AI-driven system called COiN (Contract Intelligence) that reviews legal documents and extracts key data points, significantly reducing the time and cost associated with manual processing. Similarly, Capital One uses machine learning algorithms to detect fraudulent transactions and protect customers' accounts.
Lessons Learned
Implementing data science in financial institutions is not without challenges. Common obstacles include data quality issues, integration difficulties, and resistance to change. However, by adopting a strategic approach and learning from the experiences of others, institutions can overcome these challenges. Key lessons include the importance of robust data management practices, continuous staff training, and maintaining flexibility to adapt to evolving technologies.
Expert Insights
Industry Experts Speak
Industry experts emphasize the transformative potential of data science in finance. According to Dr. Andrew Lo, a professor at MIT's Sloan School of Management, "Data science is fundamentally changing the way we understand and manage financial risks." He predicts that as data science techniques continue to evolve, their impact on the financial sector will only grow stronger.
Academic Perspectives
Academic researchers are also exploring the potential of data science in finance. Recent studies highlight the effectiveness of machine learning models in predicting stock market trends and assessing credit risk. These findings underscore the importance of continued research and collaboration between academia and industry to advance the field of data science in finance.
FAQs (Frequently Asked Questions)
What is data science, and how is it used in finance?
Data science is a multidisciplinary field that uses scientific methods, processes, algorithms, and systems to extract knowledge and insights from structured and unstructured data. In finance, data science is used for various applications, including fraud detection, risk management, customer analytics, algorithmic trading, and regulatory compliance.
How does data science improve fraud detection in banking?
Data science improves fraud detection by analyzing patterns and anomalies in transaction data. Machine learning algorithms can identify unusual activities that deviate from typical behavior, flagging them for further investigation. This helps banks prevent fraudulent transactions and protect customer assets.
What are the benefits of using data science in risk management?
The benefits of using data science in risk management include more accurate credit risk assessment, real-time risk monitoring, and enhanced decision-making. Predictive analytics allows banks to evaluate a wider range of risk factors and make informed lending decisions, reducing the likelihood of defaults.
How does data science enhance customer experiences in banking?
Data science enhances customer experiences by enabling personalized services and efficient customer support. By analyzing customer data, banks can offer tailored product recommendations and use AI-driven chatbots to provide instant, accurate responses to customer inquiries, improving overall satisfaction.
What are the challenges of implementing data science in financial institutions?
Challenges of implementing data science in financial institutions include data privacy concerns, ensuring data quality, integrating new technologies with legacy systems, and addressing the skill gap in data science expertise. Overcoming these challenges requires strategic planning, investment in infrastructure, and continuous training and development.
How is artificial intelligence used in data science for finance?
Artificial intelligence (AI) is used in data science for finance to develop predictive models, automate processes, and enhance customer interactions. AI-driven tools, such as chatbots, provide instant support, while machine learning algorithms analyze data to forecast trends and make informed decisions.
What is the future of data science in the financial sector?
The future of data science in the financial sector involves emerging trends such as predictive and prescriptive analytics, increased use of real-time data, and collaboration with fintech companies. Innovations like quantum computing and blockchain are expected to further transform the industry, offering new opportunities for efficiency and growth.
Conclusion
Data science is revolutionizing the financial and banking sectors by enhancing decision-making, improving security, increasing customer satisfaction, and reducing costs. Despite challenges such as data privacy concerns and technological barriers, the benefits of data science far outweigh the drawbacks. The latest innovations, including AI, blockchain, and quantum computing, promise to further transform the industry.
コメント