Introduction
Agriculture, one of the world's oldest industries, is witnessing a transformative shift with the integration of artificial intelligence (AI). This technological advancement is driving unprecedented efficiency, sustainability, and productivity. From precision farming to supply chain optimization, AI is set to revolutionize agricultural practices. In this blog, we will delve into the various ways AI can be leveraged in agriculture, examining its applications, benefits, and potential challenges.
Precision Farming
What is Precision Farming?
Precision farming, also known as precision agriculture, involves using technology to measure and manage variability in fields to optimize agricultural inputs and practices. By integrating AI, precision farming becomes even more effective, allowing for real-time data analysis and decision-making.
Benefits of Precision Farming with AI
Enhanced Crop Management: AI algorithms analyze data from various sources, such as satellite imagery and sensors, to provide precise recommendations on planting, watering, and harvesting.
Resource Optimization: Farmers can use AI to apply fertilizers and pesticides more efficiently, reducing waste and environmental impact.
Cost Reduction: By optimizing resource use and increasing crop yields, precision farming helps in significantly cutting down costs.
Real-World Examples:
John Deere's See & Spray: This technology uses computer vision and machine learning to identify and spray herbicides only where needed, reducing chemical use by up to 90%.
FarmLogs: An AI-driven platform that provides farmers with real-time insights into soil conditions, weather forecasts, and crop health.
Crop Monitoring and Management
AI-Powered Crop Monitoring
AI facilitates continuous crop monitoring through drones, satellites, and ground sensors. These technologies capture high-resolution images and data, which are then analyzed to assess crop health.
Key Applications
Disease Detection: AI can identify signs of diseases in crops before they become visible to the naked eye, allowing for early intervention.
Growth Tracking: By monitoring growth patterns, AI helps farmers make informed decisions about irrigation, fertilization, and harvesting.
Yield Prediction: AI models predict crop yields based on historical data and current conditions, helping farmers plan better.
Technologies Involved
Drones: Equipped with multispectral cameras, drones capture detailed images of fields, which are analyzed by AI to detect issues like nutrient deficiencies and pest infestations.
Remote Sensing: Satellites provide large-scale monitoring capabilities, with AI processing the data to offer insights on crop health and environmental conditions.
Predictive Analytics for Crop Yield
The Role of Predictive Analytics
Predictive analytics involves using historical data, statistical algorithms, and machine learning techniques to forecast future outcomes. In agriculture, predictive analytics can forecast crop yields, helping farmers make strategic decisions.
How It Works
Data Collection: Data is gathered from various sources, including weather reports, soil samples, and crop health records.
Model Training: Machine learning models are trained on this data to recognize patterns and make predictions.
Outcome Prediction: The trained models predict yields, identify potential issues, and suggest corrective actions.
Benefits
Risk Mitigation: By predicting adverse weather conditions and potential pest outbreaks, farmers can take proactive measures.
Improved Planning: Farmers can plan their planting schedules, resource allocation, and market strategies more effectively.
Case Study
IBM's Watson Decision Platform for Agriculture: This platform uses AI to analyze weather data, satellite images, and IoT sensor inputs to provide farmers with actionable insights and yield predictions.
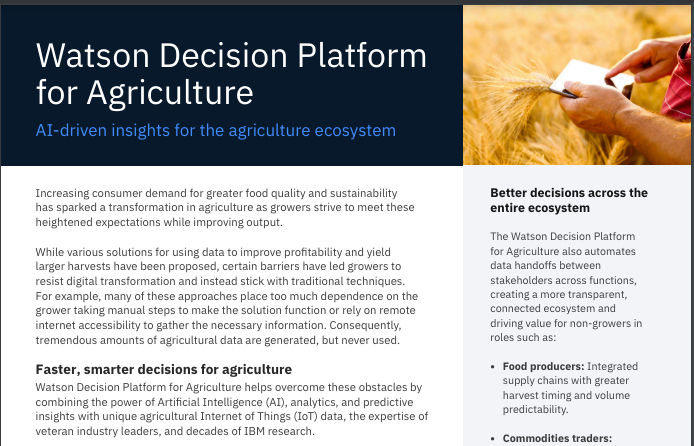
Soil Health Monitoring
Importance of Soil Health
Healthy soil is the foundation of productive agriculture. Monitoring soil health ensures optimal conditions for crop growth, leading to better yields and sustainable farming practices.
AI in Soil Monitoring
AI enhances soil health monitoring by providing detailed analyses of soil composition, moisture levels, and nutrient content.
Techniques and Tools
Soil Sensors: These devices collect data on soil temperature, moisture, pH, and nutrient levels.
AI Algorithms: Analyzing sensor data, AI algorithms offer insights into soil conditions and recommend actions to improve soil health.
Remote Sensing: Satellite imagery combined with AI helps monitor large-scale soil health, identifying areas needing intervention.
Benefits
Informed Decision Making: Farmers can make data-driven decisions about irrigation, fertilization, and crop rotation.
Sustainability: Optimizing soil health leads to sustainable farming practices, reducing the need for chemical inputs.

Pest and Disease Control
Challenges in Pest and Disease Management
Pests and diseases are major threats to agricultural productivity. Traditional methods of pest control often involve excessive use of chemicals, which can harm the environment and human health.
AI Solutions
AI offers innovative solutions for pest and disease control, improving efficiency and reducing environmental impact.
Techniques
Image Recognition: AI-powered systems use image recognition to identify pests and diseases in real-time, enabling targeted interventions.
Predictive Models: AI models predict pest outbreaks and disease spread based on weather patterns, crop conditions, and historical data.
Automated Systems: Robotics and automated systems, guided by AI, can apply pesticides or take other actions precisely where needed.
Real-World Applications
Taranis: This platform uses high-resolution imagery and AI to detect crop threats at an early stage, allowing for timely interventions.
FAO's PestLocust Monitoring System: An AI-driven tool that predicts locust outbreaks, helping farmers and governments take preventive measures.
AI in Livestock Management
Transforming Livestock Management
AI is not only beneficial for crop farming but also for livestock management, enhancing animal health, productivity, and welfare.
Key Applications
Health Monitoring: AI analyzes data from wearable devices and sensors to monitor animal health, detect illnesses early, and track vital signs.
Feed Optimization: AI algorithms optimize feeding schedules and nutrition plans based on individual animal needs and growth patterns.
Breeding Programs: AI assists in selecting the best breeding pairs to improve genetic quality and productivity.
Benefits
Increased Productivity: Healthier animals and optimized feeding lead to higher productivity and better yields.
Cost Savings: Early disease detection and efficient feeding reduce veterinary and feed costs.
Animal Welfare: Continuous monitoring ensures timely interventions, improving overall animal welfare.
Case Study
Connecterra's Ida: This AI-driven platform uses sensors and machine learning to monitor dairy cows, providing insights into health, fertility, and productivity.
Supply Chain Optimization
Importance of Supply Chain in Agriculture
An efficient supply chain is crucial for the timely delivery of agricultural products, reducing waste, and ensuring food security.
AI in Supply Chain Management
AI enhances supply chain efficiency by optimizing logistics, inventory management, and demand forecasting.
Key Applications
Demand Prediction: AI analyzes market trends and consumer behavior to forecast demand, helping farmers and distributors plan better.
Inventory Management: AI-powered systems optimize inventory levels, reducing storage costs and minimizing waste.
Logistics Optimization: AI algorithms optimize routes and schedules for transporting agricultural products, ensuring timely delivery.
Benefits
Reduced Waste: Better demand prediction and inventory management reduce food waste.
Cost Efficiency: Optimized logistics and inventory management lead to significant cost savings.
Improved Market Access: Farmers can better align their production with market demand, improving profitability.
Case Study
Kisan Network: An AI-based platform in India that connects farmers directly with buyers, optimizing the supply chain and ensuring fair prices for producers.
Challenges and Considerations
Technological Challenges
Data Quality: AI systems require high-quality data, which can be challenging to obtain in some agricultural settings.
Integration: Integrating AI with existing agricultural practices and systems can be complex and costly.
Ethical and Social Considerations
Data Privacy: The use of AI in agriculture involves collecting vast amounts of data, raising concerns about data privacy and security.
Impact on Employment: Automation and AI could potentially displace agricultural workers, necessitating strategies for workforce transition.
Economic Factors
Cost of Implementation: The initial investment in AI technology can be high, posing a barrier for small-scale farmers.
Return on Investment: Farmers need to see clear benefits and ROI to justify the adoption of AI technologies.
Future of AI in Agriculture
Trends and Innovations
Increased Adoption: As technology becomes more affordable and accessible, more farmers are expected to adopt AI solutions.
Integration with Other Technologies: AI will increasingly integrate with IoT, blockchain, and other technologies to enhance agricultural practices.
Sustainability Focus: AI will play a crucial role in promoting sustainable farming practices, helping to address global challenges like climate change and food security.
Potential Impact
Food Security: AI can help increase agricultural productivity, ensuring food security for a growing global population.
Environmental Conservation: By optimizing resource use and reducing chemical inputs, AI contributes to environmental conservation.
Economic Growth: Enhanced agricultural productivity and efficiency can drive economic growth, particularly in rural areas.
Frequently Asked Questions (FAQs)
How is AI used in precision farming?
AI in precision farming involves using data from satellites, sensors, and drones to optimize agricultural practices. This helps in making precise recommendations for planting, watering, and harvesting, enhancing crop management and resource efficiency.
What are the benefits of AI-powered crop monitoring?
AI-powered crop monitoring helps in early disease detection, growth tracking, and yield prediction. It enables farmers to make informed decisions about irrigation, fertilization, and harvesting, improving crop health and productivity.
How does AI help in pest and disease control?
AI aids in pest and disease control through image recognition and predictive models. It identifies pests and diseases in real-time, enabling targeted interventions and predicting outbreaks based on weather patterns and crop conditions.
What are the challenges of implementing AI in agriculture?
Challenges include ensuring data quality, integrating AI with existing practices, addressing data privacy concerns, managing the impact on employment, and the high cost of initial implementation. Overcoming these challenges is essential for the successful adoption of AI in agriculture.
What is the future of AI in agriculture?
The future of AI in agriculture includes increased adoption, integration with other technologies like IoT and blockchain, and a focus on sustainability. AI will play a crucial role in enhancing food security, environmental conservation, and economic growth, particularly in rural areas.
Conclusion
Artificial intelligence is poised to revolutionize agriculture, offering numerous benefits across various aspects of farming. From precision farming and crop monitoring to predictive analytics and livestock management, AI enhances efficiency, productivity, and sustainability. By leveraging advanced technologies, farmers can optimize resource use, reduce environmental impact, and improve crop yields.
However, the adoption of AI in agriculture also presents challenges, including technological, ethical, and economic considerations. Ensuring data quality, addressing privacy concerns, and managing the cost of implementation are critical for successful integration. Despite these challenges, the future of AI in agriculture looks promising, with ongoing advancements and increasing accessibility.
Comments